Day :
- Plenary Session
Session Introduction
Karmen Kern Pipan
Ministry of Public Administration, Slovenia
Title: Big data – Big challenge for public administration – Experiences of pilot project in Ministry of Public Administration of Republic Slovenia
Time : 11:20-11:50
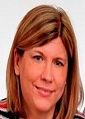
Biography:
Karmen Kern Pipan started her career in the private sector in the field of Informatics and Telecommunications and later continued in public administration for different positions at Metrology Institute of Republic Slovenia as Quality Manager and Head of Department for Quality and Business Excellence. Currently, she works as Secretary and Project Manager at Ministry of Public Administration of Republic Slovenia in IT Directorate. As an expert, she is involved in the field of Data Management, Business Intelligence and Big Data Analytics to improve data based decision making at Slovenian Public Administration. She collaborates a lot with successful Slovenian companies and international professional sphere to identify good practices in this field. She leads an inter-ministerial task force for the preparation of the Public Administration Development Strategy 2015-2020. She has published several papers in reputed national and international conferences and journals.
Abstract:
The pilot project - Big data analysis for HR efficiency improvement has been established as part of the development oriented strategy supporting ICTs as an enabler of development of data driven public administration in Republic Slovenia. It has been run within Ministry of Public Administration of Republic Slovenia in collaboration with EMC Dell as an external partner. This pilot project has been launched aiming to learn what big data tool installed on Slovenian State Cloud Infrastructure could enable in terms of the research of HR data of our ministry to improve our efficiency. Therefore, anonymized internal data sources containing time management, HR database, finance database and public procurement had been combined with external resources using postal codes of employees and weather data to identify potentials for improvement and possible patterns of behavior. The results showed that there is considerable potential for improvement in the field of HR and lowering costs in the field of public procurement within our ministry.
Boris Mirkin
Higher School of Economics,Russia
Title: A Complementary Square-Error Clustering Criterion and Initialization of K-Means
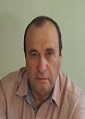
Biography:
Boris Mirkin holds a PhD in Computer Science and DSc in Systems Engineering degrees from Russian Universities. He published a dozen monographs and a hundred refereed papers. In 1991-2010 he extensively traveled taking visiting research appointments in France, USA, Germany, and a teaching appointment at Birkbeck University of London, UK. He develops methods for clustering and interpretation of complex data within the “data recovery” perspective. Currently these approaches are being extended to automation of text analysis including use of hierarchical ontologies.
Abstract:
Clustering is a set of major data analysis techniques. The square-error clustering criterion underlies most popular clustering methods including k-means partitioning and Ward agglomeration. For the k-means, the square-error criterion to be minimized is the sum of squared Euclidean distances from all the objects to their respective cluster centers/means, W(S,c), where S is the sought partition of the set of objects and c is the set of within-cluster means. The method’s popularity stems from the simplicity of computation and interpretation. Yet there is a catch: the user is to specify both the number of clusters and the initial locations of cluster centers, which can be of an issue sometimes. To tackle the problem, the current author proposes using the complementary criterion. It is not difficult to prove that there is a complementary criterion, B(S,c), to be maximized, such that W(S,c)+B(S,c)=T where T is the data scatter. The complementary criterion B(S,c) is the sum of individual cluster contributions, equal each to the product of the cluster’s cardinality and the squared Euclidean distance from the cluster’s center to 0. Therefore, the complementary criterion leads to a set of anomalous clusters, which can be found either one-by-one or in parallel. Our experiments show that methods emerging in this perspective are competitive, and frequently superior, to other initialization methods.
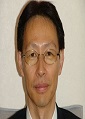
Biography:
Kazumi Nakamatsu received the Dr. Sc. from Kyushu University, Japan. He is a Professor at University of Hyogo, Japan. He contributed over 150 journal/conference papers and book chapters, and edited/authored 12 books published by prominent publishers. He has chaired various international conferences/workshops, and he has been a program committee member/chair of academic conferences. He serves as Editor-in-Chief of the Int’l J. Reasoning-based Intelligent Systems and an editorial member/associate editor of many international journals. He has contributed numerous invited lectures at conferences/academic organizations. He received some conference/best paper awards at some international conferences.
Abstract:
Paraconsistent logic is a well-known formal logic that can deal with contradiction in the framework of a logical system consistently. One of paraconsistent logics called annotated logic was proposed by Prof. Newton da Costa et al. and its logic program was been developed by Prof. V.S. Subrahmanian et al. later as a tool of dealing with data in knowledge bases. Some years later a kind of paraconsistent annotated logic program has been developed for dealing with non-monotonic reasoning such as default reasoning by Kazumi Nakamatsu. Recently a paraconsistent annotated logic program called Extended Vector Annotated Logic Program with Strong Negation (abbr. EVALPSN) that can deal with conflict resolving, defeasible deontic reasoning, plausible reasoning, etc. has been developed and already been applied to various intelligent controls and safety verification systems such as pipeline valve control, traffic signal control, railway interlocking safety verification, etc. Furthermore, most recently one specific version of EVALPSN called Before-after EVALPSN (abbr. Bf-EVALPSN) that can deal with before-after relations between processes (time intervals) has been developed.
In this lecture, I introduce how EVALPSN and Bf-EVALPSN deal with contradictory data with a small example and can be applied to intelligent control or safety verification of sensed data.
Pavel Kisilev
Toga Networks Ltd., Israel
Title: Smart data acquisition and analysis for deep learning based systems
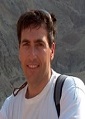
Biography:
Pavel Kisilev serves as the CTO of Artificial Intelligence at Huawei Research Center in Israel. He completed his Graduation at Technion, Israel Institute of Technology in 2002 with a PhD in Electrical Engineering. Before joining Huawei, he was a Lead Scientist at IBM Research from 2011-2016, a Senior Research Scientist at HP Labs from 2003-2011, and a Research Associate at Technion. His research interests include computer vision, deep learning, general statistical methods, and inverse problems. He is an author of over 50 filed patents, three book chapters, and of nearly 50 papers in top journals and conferences in computer science.
Abstract:
Deep Learning (DL) becomes the method of choice in many fields of computer science, including computer vision, Natural Data Processing (NLP), autonomous systems, and many others. While DL methods are known for their superior performance, in order to achieve it, large amount of training examples are required. Furthermore, the quality of training examples affects largely the performance of DL training and learnt model quality. In particular, if the training examples represent well the real world phenomena, a good model is expected to learn. If, on the other hand, the examples are highly correlated and represent only sparse knowledge about phenomena, the learnt model quality will be low. In this talk, we present several general principles and methods to diagnose the data quality, and also the suitability of DL architecture to model the data at hand. We also propose several methods to pre-process raw data to better suit the requirements of DL systems. We show several examples of applications of our framework to various datasets, including known large image datasets with millions of images, binary sequence sets, gene datasets, and others. We show the efficacy of the proposed methods to analyze and predict the performance of DL methods on a given data.
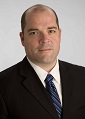
Biography:
Bennett B Borden is a Chief Data Scientist at Drinker Biddle & Reath. He is a globally recognized authority on the legal, technology and policy implications of information. His ground-breaking research into the use of machine-based learning and unstructured data for organizational insight is now being put to work in data-driven early warning systems for clients to detect and prevent corporate fraud and other misconduct. He received his Master of Science in Data Analytics at New York University and his JD at Georgetown University.
Abstract:
Corporate misconduct costs $3 Trillion worldwide every year to prevent, detect and deal with its consequences. If we can predict when someone will purchase a product, click on an ad, or how they will vote for a candidate, why can’t we predict when he or she will engage in some form of fraud or other misconduct? Well, perhaps we can. In this session, Chief Data Scientist Bennett Borden, from the law firm Drinker Biddle & Reath will present his work on developing algorithms to predict corporate misconduct and how this technology is being used today and how it will likely be used in the future.
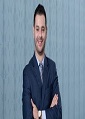
Biography:
Nick Freris is an Assistant Professor of Electrical and Computer Engineering (ECE), and Director of Cyber-physical Systems Laboratory (CPSLab) at New York University Abu Dhabi. He received Diploma in ECE at National Technical University of Athens in 2005; MS degree in ECE in 2007; MS in Mathematics in 2008 and; PhD in ECE at University of Illinois at Urbana-Champaign. His work was recognized with 2014 IBM High Value Patent award. He is a senior member of IEEE, and member of SIAM and ACM.
Abstract:
Big data pertain to multiple facets of modern science and technology enlisting biology, physics, social networks, financial analysis, smart cities and many more. Despite the overwhelming amount of accessible data alongside the abundance of mining schemes, the prelude of data mining faces a key challenge in that the data are hardly ever available in their original form. Common operations such as compression, anonymization and right protection may significantly affect the accuracy of the mining outcome. We will discuss the fundamental balance between data transformation and data utility under prevalent mining operations such as search, K-nearest neighbors and clustering. In specific, we will illustrate classes of data transformation – information extraction methods where it is actually feasible to acquire the exact mining outcome even when operating on the transformed domain. This talk will feature three specific problems: Optimal distance estimation of compressed data series; nearest neighbor preserving watermarking and; cluster preserving compression. We provide provable guarantees of mining preservation, and further highlight the efficacy and efficiency of our proposed methods in a multitude of datasets: weblogs, VLSI images, stock prices, videos, and images from anthropology, natural sciences, and handwritings.
- Plenary Session
Session Introduction
Fairouz Kamareddine
Heriot-Watt University, UK
Title: Computerizing mathematical text, debugging proof assistants and object versus metal level
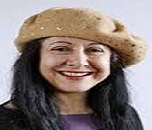
Biography:
Fairouz Kamareddine has been involved in a number of worldwide consultancy assignments, especially on education and research issues working for United Nations and the EU. Her research interest includes Interface of Mathematics, Logic and Computer Science. She has held numerous invited positions at universities worldwide. She has well over 100 published articles and a number of books. She has played leading roles in the development, administration and implementation of interdisciplinary, international, and academic collaborations and networks.
Abstract:
Mathematical texts can be computerized in many ways that capture differing amounts of the mathematical meaning. At one end, there is document imaging, which captures the arrangement of black marks on paper, while at the other end there are proof assistants (e.g., Mizar, Isabelle, Coq, etc.), which capture the full mathematical meaning and have proofs expressed in a formal foundation of mathematics. In between, there are computer type setting systems (e.g., LATEX and presentation MathML) and semantically oriented systems (e.g., Content MathML, OpenMath, OMDoc, etc.). In this talk, we advocate a style of computerization of mathematical texts which is flexible enough to connect the different approaches to computerization, which allows various degrees of formalization, and which is compatible with different logical frameworks (e.g., set theory, category theory, type theory, etc.) and proof systems. The basic idea is to allow a man-machine collaboration which weaves human input with machine computation at every step in the way. We propose that the huge step from informal mathematics to fully formalized mathematics be divided into smaller steps, each of which is a fully developed method in which human input is minimal. We also propose a method based on constraint generation and solving to debug proof assistants written in SML and reflect on the need and limitation of mixing the object and meta-level.
Bennett B. Borden
Drinker Biddle & Reath, Washington, DC
Title: Big Data Winners and Losers: The Ethics of Algorithms
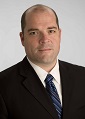
Biography:
Bennett B. Borden is a partner at Drinker Biddle & Reath and its Chief Data Scientist, the only Chief Data Scientist who is also a practicing attorney. Bennett is a globally recognized authority on the legal, technology and policy implications of information. Bennett’s ground-breaking research into the use of machine-based learning and unstructured data for organizational insight is now being put to work in data-driven early warning systems for clients to detect and prevent corporate fraud and other misconduct. Bennett received his Masters of Science in Data Analytics at New York University and his JD from Georgetown University.
Abstract:
Analytic models are playing an increasing role in the development, delivery and availability of goods and services. Who gets access to what goods or services and at what price are increasingly influenced by algorithms. This may not matter when we’re talking about a $0.25 coupon for a candy bar, but what about public goods and services like education, healthcare, and energy distribution? What about predicting who will get a job or how we will police our society? In this session, we will explore the socioeconomic impact of algorithms, the ethics of big data, and how to work ethics into our analytics projects.
Sumit Pal
Independent Consultant (Architect, Developer) Big Data & Data Science, USA
Title: SQL On Big Data – Technology, Architecture and Innovations
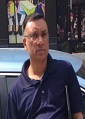
Biography:
Sumit has more than 22 years of experience in the Software Industry in various roles spanning companies from startups to enterprises.He is a big data, visualisation and data science consultant and a software architect and big data enthusiast and builds end-to-end data-driven analytic systems.Sumit has worked for Microsoft (SQL server development team), Oracle (OLAP development team) and Verizon (Big Data analytics team) in a career spanning 22 years.Currently, he works for multiple clients advising them on their data architectures and big data solutions and does hands on coding with Spark, Scala, Java and Python.Sumit has spoken at Big Data Conferences in Boston, Chicago, Las Vegas and Vancouver.He has extensive experience in building scalable systems across the stack from middletier, data tier to visualization for analytics applications, using BigData, NoSQL DB. Sumit has deep expertise in DataBase Internals, Data Warehouses, Dimensional Modeling, Data Science with Scala, Java and Python and SQL.Sumit started his career being part of SQLServer Development Team at Microsoft in 1996-97 and then as a Core Server Engineer for Oracle Corporation at their OLAP Development team in Boston, MA, USA.Sumit has also worked at Verizon as an Associate Director for Big Data Architecture, where he strategized, managed, architected and developed platforms and solutions for analytics and machine learning applications.Sumit has also served as Chief Architect at ModelN/LeapfrogRX (2006-2013)- where he architected the middle tier core Analytics Platform with open source olap engine (Mondrian) on J2EE and solved some complex Dimensional ETL, Modelling and performance optimization problems.
Abstract:
With the rapid adoption of Hadoop in the enterprise it has become all the more important to build SQL Engines on Hadoop for all kinds of workloads for almost all kind of end users and use cases. From low latency analytics based SQL to ACID based semantics on Hadoop for Operational Systems, to SQL for handling unstructured and streaming data, SQL is fast becoming the ligua-franca in the big data world too. The talk focuses on the exciting tools, technologies and innovations and their underlying architectures and the exciting road ahead in this space. This is a fiercely competitive landscape with vendors and innovators trying to capture mindshare and piece of the pie – with a whole suite of innovations like – index based SQL solutions in Hadoop to OLAP with Apache Kylin and Tajo to BlinkDB and MapD.
Topics :
- Why SQL on Hadoop
- Challenges of SQL on Hadoop
- SQL on Hadoop Architectures for Low Latency Analytics ( Drill, Impala, Presto, SparkSQL, JethroData)
- SQL on Hadoop Architecture for Semi-Structured Data
- SQL on Hadoop Architecture for Streaming Data and Operational Analytics
- Innovations ( OLAP on Hadoop, Probabilistic SQL Engines, GPU Based SQL Solutions )
Bertrand Hassani
University College London, UK
Title: Regulatory learning; what framework can be proposed to ensure that the financial environment can be controlled?
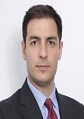
Biography:
Bertrand Hassani is an Associate Researcher at Paris 1 University and University College London. He wrote several articles dealing with Risk Measures, Data Combination, Scenario Analysis and Data Science. He was the Global Head of Research and Innovations of Santander Group for the risk division and is now the Chief Data Scientist of Capgemini Consulting. In this role, he aims at developing novel approaches to measure risk (financial and non-financial), to know the customers, to improve predictive analytics for supply chain (among others) relying on methodologies coming from the field of data science (data mining, machine learning, A.I., etc.).
Abstract:
The arrival of big data strategies is threatening the latest trends in financial regulation related to the simplification of models and the enhancement of comparability of approaches chosen by the various entities. Indeed, the intrinsic dynamic philosophy of big data strategies is almost incompatible with the current legal and regulatory framework in particular the one related to model risk governance. Besides, as presented in our application to credit scoring, the model selection may also evolve dynamically forcing both practitioners and regulators to develop libraries of models, strategies allowing to switch from one to the other and supervising approaches allowing financial institutions to innovate in a risk mitigated environment. The purpose of this paper is therefore to analyse the issues related to the big data environment and in particular to machine learning models and to propose solutions to regulators, analyzing the features of each and every algorithm implemented, for instance a logistic regression, a support vector machine, a neural network, a random forest and gradient boosting.
Pierre Hansen
GERAD and HEC Montréal,Canada
Title: Two applications of Variable Neighborhood Search in Data Mining
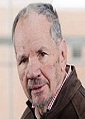
Biography:
Pierre Hansen is professor of Operations Research in the department of decision sciences of HEC Montréal. His research is focussed on combinatorial optimization, metaheuristics and graph theory. With Nenad Mladenovic, he has developed the Variable Neighborhood Search metaheuristic, a general framework for building heuristics for a variety of combinatorial optimization and graph theory problems. Pierre Hansen has received the EURO Gold Medal in 1986 as well as other prizes. He is a member of the Royal Society of Canada and the author or co-author of close to 400 scientific papers. His first paper on VNS was cited almost 3000 times.
Abstract:
Many problems can be expreesed as global or combinatorial optimization problems, however due the vast increase in the availability of data bases, realistically sized instances cannot be solved in reasonnable time. Therfore, one must be often content with approximate solutions obtained by heuristics. These heuristics can be studied systematically by some general frameworks or metaheuristics (genetic search, tabu search, simulated annealing, neuron networks, ant colonies and others). Variable Neighborhood Search (VNS) proceeds by systematic change of neighborhoods bith in the descent phase towards a local minimum and in a perturbation phase to get out of the corresponding valley. VNS heuristics have been developed for many classical problems such as TSP, quadratic assignment, p-median, and others. Instances of the latter problem with 89600 entities in the Euclidean plane have been solved with an ex-post error not larger than 3%.
In the last 2 decades, several discovery systems for graph theory have been proposed (Cvetkovic's Graph; Fajtlowicz's Graffiti; Caporossi and Hansen's AutoGraphiX (AGX)). AGX uses VNS to find systematically extremal graphs for many conjectures judged to be of interest. Aouchiche systematically studied relations between 20 graph invariants taken by pairs, considering the four basic operations (-, +, /, x). Conjectures were found in about 700 out of 1520 cases. A majority of these 1520 cases were easy and solved automatically by AGX. Examination of the extremal graphs found suggests open conjectures to be solved by graph theoretical means. This led to several tens of papers by various authors mainly from Serbia and from China.
Parameshwar P Iyer
Indian Institute of Science, India
Title: From knowledge to wealth: Intellectual property and technology licensing
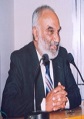
Biography:
Parameshwar P Iyer holds a Bachelore degree from IIT Kharagpur, Master degree from University of Illinois, and a Doctorate from University of California. He has over 43 years of teaching, research, and consultancy experience in Engineering and Technology Management. He has held several important academic and administrative positions, including being the Founder Professor of an IIM in India. He has over 50 publications and four books to his credit. He has received several professional awards, including a prestigious Fellowship from Unversity of Illinois. Currently, he is a Principal Research Scientist at Indian Institute of Science.
Abstract:
The Office of Intellectual Property and Technology Licensing (IPTeL) at the Indian Institute of Science is geared towards identifying and sourcing the IP at IISc, facilitating the steps towards protection of the IP, continuously safeguarding and prosecuting the IP, and finally enabling the commercial and social utilization of the IP, through appropriate means of licensing and technology transfer.
The specific activities and roles of IPTeL are as follows:
• Identifying the sources of Intellectual Property at the Indian Institute of Science;
• Inviting Invention Disclosures from the Inventor(s);
• Evaluation and Assessment of the above Invention Disclosure(s);
• Assisting the Inventor(s) with prior art search;
• Assigning appropriate Patent Attorney(s) to assist the Inventor(s) in drafting claims and specifications for the Invention(s);
• Informing the Inventor(s) of the various stages of progress during the filing, prosecution, and maintenance of the Intellectual Property;
• Assisting in the efforts of the Inventor(s) towards Technology Licensing, and other forms of Technology Transfer to bring the Invention to “commercial” and/or “social” practice.
Some of the interesting “inventions” from the Institute, and the accompanying “technology transfer” practices are highlighted in this paper. These include:
• Portable Washing Machine for Rural Areas
• Flood-resistant Septic Tank
• Optical nano sensor
• Electrical Gradient Augmented Fluid Filtration Apparatus
• Patient Transfer Device
• Electrochemical Test Cell
Nada Philip
Kingston University London, UK
Title: Big data analytics and visualization for healthcare – A glance into the AEGLE platform features via its presentation layer
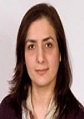
Biography:
Nada Philip is an Associate Professor in the field of Mobile Health at Kingston University London. She is the Founder of Digital Media for Health Research Group. She has been involved in many national and international mHealth projects in the areas of “Chronic disease management (e.g. diabetes, COPD and Cancer), big data analytics for health, decision support systems, telehealth and telecare in developing countries, social robotics, medical video streaming, namely; OTELO, Delphi, LTE-Health, WELCOME and AEGLE”. She is the author and co-author of more than 50 journals, peer reviewed conferences and book chapters. She is a member of the review panels for many grants bodies, transactions, and conferences.
Abstract:
Recently there has been great interest in health data and on how to produce value out of it so that it can support optimized integrated clinical decision making about the health of individuals and reduce cost. Many initiatives exist at the European level and worldwide, pinpointing the importance and usefulness of healthcare big data. One of these initiatives is the AEGLE EU project which aims to generate value from the healthcare data value chain data with the vision to improve translational medicine and facilitate personalized and integrated care services and thus improving overall healthcare at all levels, to promote data-driven research across Europe and to serve as an enabler technology platform, enabling business growth in the field of big data analytics for healthcare. In this talk, we will be looking into the main technical features of the AEGLE platform through its presentation layer that act as the user interface to the Big Data analytics and visualization infrastructure and algorithms of the AEGLE platform. In particular, we will be looking into the type 2 diabetes (T2D) use case and present some of the initial results of scenarios that aims at using analytics and visualization tools to discover knowledge and the causing factors of diabetes complications and their correlation to medication modality as well as biological markers while providing the potential to act as a predictive tool of such complications.
- Data Mining Applications in Science, Engineering, Healthcare and Medicine | Data Mining Methods and Algorithms | Artificial Intelligence | Big Data Applications | Big Data Algorithm | Data Privacy and Ethics | Data Mining Analysis | Business Analytics | Optimization and Big Data | New visualization techniques | Clustering
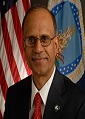
Chair
Michael Valivullah
NASS, USA
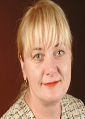
Co-Chair
Petra Perne
Institute of Computer Vision and applied Computer Sciences, Germany
Session Introduction
Tommi Kärkkäinen
University of Jyväskylä, Finland
Title: Scalable robust clustering method for large and sparse data
Time : 15:00-15:20
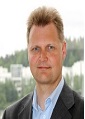
Biography:
Tommi Kärkkäinen has completed his PhD at University of Jyväskylä in 1995 and worked as a Full Professor in the Faculty of Information Technology since 2002. He has been and is serving in many positions of administration and responsibility at the faculty and the university level. He has published over 150 research papers, led dozens of R&D projects, and supervised over 20 PhD theses.
Abstract:
Clustering is the most common unsupervised, descriptive analysis technique to reveal hidden patterns and profiles from a dataset. There exists large number of different clustering algorithms, but approaches that specifically address clustering of sparse datasets are still scarce, even if real world datasets are many times characterized by missing values with unknown sparsity pattern. Typical approaches in the knowledge discovery process is to either completely omit the observations with missing values or use some imputation method to fill in the holes of data. However, the throw data away approach does not utilize all possible data and the imputation necessarily introduces assumptions about the unknown density of the data. Moreover, by the well-known curse-of-dimensionality results, such assumptions are no more valid in the high dimensional spaces. The purpose of this presentation is to describe and summarize a line of research that addresses the sparse clustering problems with the available data strategy and robust prototypes. The strategy allows one to utilize all available data without any additional assumptions. The actual prototype-based clustering algorithm, the k-spatial medians, relies on the computation of a robust prototype as cluster centroid, again argumenting on non-Gaussian within-cluster error in comparison to the classical k-means method. As with any prototype-based algorithm, the initialization step of the locally improving relocation algorithm has an important role and should be designed to handle the sparse data. Such an approach is proposed and the scalability of a distributed implementation of the whole algorithm is tested with openly available large and sparse datasets.
Fred Jacquet
Product Marketing Manager | Big Data Analytics - Modern Data Platform
Title: Data is not wrong, but your use of it might be
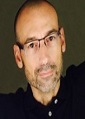
Biography:
Fred Jacquet has over 20 years’ experience in the IT industry, working in Evangelist, CTO and Architect roles within a variety of leading data-driven companies.His main area of expertise is in Business Intelligence, Data Integration and Big Data. He is committed to helping organizations on their mission to become successfully data driven through evangelization, education and enablement.
Abstract:
Some companies are born in the Data Intelligence Era, some need to redefine their IT
infrastructure to stay competitive in the world of improved analytics, scale, speed, production
and economics.The old data warehousing and Business Insights tools helped find the answers to “ What happened?” but are not able to answer the new questions asked by more and more forward thinking companies. Traditional warehousing tools such as ETL, RBMS and OLAP databases can not provide answers to questions such as “What’s happening right now?” or “What will happen?”. The new Era of Analytics demands speed, scale and reduced costs from every IT team. This presentation will take you through the considerations and steps of modernizing your data warehouse to become ready for Big Data Analytics and evaluate if the Data Lake is right for you and your business needs. Don’t be one of the many companies that failed to grasp this opportunity to leapfrog their competition. After all, over 50% of companies that were originally on the Fortune 500 list have vanished since 2000, because their failed to innovate and keep up with the changes in the market.
Morgan C. Wang
University of Central Florida, USA
Title: An Automatic Data Prediction System with Business Applications
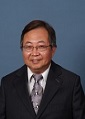
Biography:
Morgan C. Wang received his Ph.D. from Iowa State University in 1991. He is the funding Director of Data Mining Program and Professor of Statistics at the University of Central Florida. He has published one book (Integrating Results through Meta-Analytic Review Using SAS Software, SAS Institute, 1999), and over 80 papers in referee journals and conference proceedings on topics including interval analysis, meta-analysis, computer security, business analytics, health care analytics and data mining. He is the elected member of International Statistical Association and member of American Statistical Association and International Chinese Statistical Association.
Abstract:
An automatic prediction model building system was developed. This system has five components: data exploration component, data preparation component, model building component, model validation and selection component, and the result automatic generation component. All components are resident inside the data warehouse and can be used by compony personal without model building training. A case study using this system on solving a insurance firm in china will be discussed in this presentation as well.
Witold Dzwinel
AGH University of Science and Technology, Poland
Title: A novel linear-time and memory saving approach for visual exploratory data analysis: data embedding and graph visualization
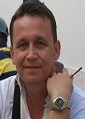
Biography:
Witold Dzwinel holds Full Professor position at AGH University of Science and Technology, Department of Computer Science in Krakow. His research activities focus on “Computer modeling and simulation methods employing discrete particles”. Simultaneously, he is doing research in interactive visualization of big data and machine learning algorithms. He is the author and co-author of about 190 papers in computational science, computational intelligence and physics.
Abstract:
Data embedding (DE) and graph visualization (GV) methods are very congruent tools used in exploratory data analysis for visualization of complex data such as high-dimensional data and complex networks, respectively. However, high computational complexity and memory loads of existing DE and GV algorithms (based on t-SNE concept from one hand, and force-directed methods from the other), considerably hinders visualization of truly large and big data consisting of as many as M~106+ data objects and N~103+ dimensions. In this paper, we demonstrate the high computational efficiency and robustness of our approach to data embedding and interactive data visualization. We show that by employing only a small fraction of distances between data objects, one can obtain very satisfactory reconstruction of the topology of N-D data in 2D in a linear-time O (M). The IVHD (Interactive Visualization of High-Dimensional Data) method quickly and properly reconstructs the N-D data topology in a fraction of computational time required for the state-of-art DE methods such as bh-SNE and all its clones. Our method can be used for both metric and non-metric (e.g. large graphs) data visualization. Moreover, we demonstrate that even poor approximations of the nearest neighbor (NN) graph, representing high-dimensional data, can yield acceptable data embedding. Furthermore, some incorrectness in the nearest neighbor list can often be useful to improve the quality of data visualization. This robustness of IVHD, together with its high memory and time efficiencies, meets perfectly the requirements of big and distributed data visualization, when finding the accurate nearest neighbor list represents a great computational challenge.
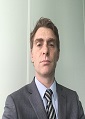
Biography:
Nicola Wesner has completed his PhD in Economics at Paris X Nanterre University in 2001 and he is an Associate Actuary since 2011. He is the Head of the Pension department at Mazars Actuariat, an audit and advisory firm. He has published many papers in reputed journals and has specialized periodicals on various subject such as econometrics, quantitative finance, insurance and pension, and data mining.
Abstract:
This paper presents a very simple and intuitive multi-objective optimization method that makes use of interactive visualization techniques. This approach stands mid-way between the brush and link technique, a visual method used in operational research for exploratory analysis of multidimensional data sets and interactive multi-criteria decision methods that use the concept of reference point. Multiple views of the potential solutions on scatterplots allow the user to directly search acceptable solutions in bi-objective spaces whereas a Venn diagram displays information about the relative scarcity of potential acceptable solutions under distinct criteria. Those very intuitive data visualization techniques allow for comprehensive interpretation and permit to communicate the results efficiently. More generally the combination of information visualization with data mining allows the user to specify what he is looking for, yields easily reportable results and respects human responsibility. An application to the visual steering of genetic algorithms in a multi-criteria strategic asset allocation optimization problem is presented.
S.N.Mohanty
KIIT University, India
Title: Multi-criteria Decision-making for Purchasing Cell Phones Using Machine Learning Approach
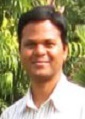
Biography:
Prof. Dr. S.N.Mohanty, received his PhD from IIT Kharagpur, India in the year 2014, with MHRD scholarship from Govt of India. He has recently joined as Asst. Professor in the School of Computer Science & Engineering at KIIT University. His research areas include Data mining, Big Data Analysis, Cognitive Science, Fuzzy Decision Making, Brain-Computer Interface, Cognition, and Computational Intelligence. Prof. S N Mohanty has received 2 Best Paper Awards during his PhD at IIT Kharagpur from International Conference at Benjing, China, and the other at International Conference on Soft Computing Applications organized by IIT Rookee in the year 2013. He has published 5 International Journals of International repute and has been elected as Member of Institute of Engineers and IEEE Computer Society. He also the reviewer of IJAP, IJDM International Journals.
Abstract:
The process of cell-phone selection for purchasing is a multi-criteria decision-making (MCDM) problem with conflicting and diverse objective. In this study, discusses various techniques using machine learning approach. To begin, participants responded to a questionnaire having different latest features available in a cell-phone. Seven independent input variables cost, talk-time, rear camera, weight, size, memory and operating system, where then derive from participants respondents. Linguistic terms such as low, medium and high were used to represent each of the input variables. Using Mamdani approach both traditional fuzzy reasoning tool (FLC) and neuro-fuzzy system (ANFIS) were designed for three input and one output process. The neuro-fuzzy system was trained using a back-propagation algorithm. Compare two traditional fuzzy reasoning tool, artificial neural network approach (ANN) the neuro-fuzzy system could provide better accuracy for selecting a cell-phone for personal use.
Jenny Lundberg
Linnaeus University, Sweden
Title: Big data applied in healthcare context: The case study of diabetes
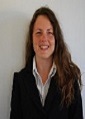
Biography:
Jenny Lundberg has completed her PhD in Computer Science at 2011 at BTH, the most profiled IT University in Sweden. She is a Senior Lecturer at Linnaeus University and a Researcher at Lund University. She has extensive international research and education collaboration experiences. Her research interest is in health applications and work in close cooperation with clinical researchers in healthcare with Big data, e- and m-health approaches and techniques.
Abstract:
As the industry 4.0 era gives us extensive IoT opportunities to provide evidence and context based data, opening for new approaches and methods to meet societal challenges. The handling of chronic diseases is global and poses challenges to the current health systems. The incidence of the chronic disease diabetes is of epidemic character. In 2015, 415 million in the world have diabetes and it is estimated that by 2040, 642 million in the world will have diabetes. More specifically: Diabetes is a heterogeneous group of conditions that all result in, and defined by, rise in plasma glucose level if not well treated. If it is untreated, death is sooner or later, if later with a lot of unpleasant complications over time. There are two main types of diabetes, type 1 (10% of all); and type 2 (85-90% of all). Diabetes places extremely high demands on the individual in terms of self-care, and a lot of complications can occur. It is a well-known fact that this creates serious health condition and high social costs. Potentially, this can be prevented with new methods for better support for self-care. Given developments, recent advances in mobile computing, sensor technology, big data can be used to better understand diabetes, measurements and data. To overcome some of the problems in this area, open data, such as social media, special designed apps, sensors and wearables can be used to find proactive ways and methods of diabetes treatment.
- Data Mining Applications in Science, Engineering, Healthcare and Medicine | Data Mining Methods and Algorithms | Data Mining Tools and Software | Big Data Applications | Data Mining Tasks and Processes | Data Privacy and Ethics | Big Data Technologies | Social network analysis | Business Analytics | Search and data mining | Clustering
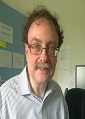
Chair
Fionn Murtagh
University of Huddersfield, UK
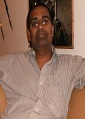
Co-Chair
Narasimha Murty Yedidi
Electronic Arts, USA
Session Introduction
Knut Hinkelmann
FHNW University of Applied Sciences and Arts Northwestern, Switzerland
Title: An innovation framework for big data
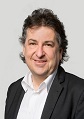
Biography:
Knut Hinkelmann is Professor for Information Systems and Head of the Master of Science in Business Information Systems at the FHNW University of Applied Sciences and Arts Northwestern Switzerland. He is a Research Associate at the University of Pretoria (South Africa) and Adjunct Professor at the University of Camerino (Italy). Prior to that he has worked at the German Research Center for Artificial Intelligence (DFKI) and as Product Manager Innovation for Insiders Information Management
Abstract:
Big data has challenged existing business models and provided new business opportunities. Companies capture large volume and variety of transactional data, which contains information about their customers, suppliers and operations. Advanced data analysis techniques can help to gain knowledge about patterns, trends and user behaviors from such large datasets. This knowledge empowers businesses to innovate new products, services and business models. Scientific literature discusses use cases of value creation, data analysis techniques and technologies supporting big data. According to recent studies, the main challenge faced by companies is the proper utilization of the knowledge extracted via data analysis to create meaningful innovations. The current innovation frameworks like Google’s Design Sprint guide organizations to create innovative IT applications in an agile manner. Design thinking oriented innovation frameworks like the one from Beckman and Barry (2007) place a strong emphasis on observations, e.g. to understand customer behavior and to identify their (implicit) needs. In today’s digitalized world, however, the observation of such behavior requires analyzing digital traces of on-line transactions and combining it with data from different sources. We therefore propose to develop an innovation framework for big data that helps companies to exploit the knowledge generated from such data present within or outside the organization. This framework will provide the best practices, data analysis tools and technologies that can guide the companies to innovate from big data. In order to give meaning to the identified patterns, the data analysis is combined with background knowledge represented in ontology.
Alla Sapronova
Uni Research Computing, Norway
Title: Prune the inputs, increase data volume, or select a different classification method – a strategy to improve accuracy of classification
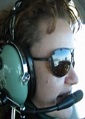
Biography:
Dr. Alla Sapronova has completed her PhD at the age of 29 years from Moscow State University, Russia and postdoctoral studies from UniFob, University of Bergen, Norway. She is the Head of Data Science at Center for Big Data Analysis, Uni Research, a multidisciplinary research institute in Bergen, Norway. Last 5 years she has published more than 15 papers in reputed journals and has been serving as an external censor for University of Bergen, Norway and Nelson Mandela Municipal University, South Africa.
Abstract:
Classification, the process of assigning data into labeled groups, is one of the most common operation in data mining. Classification can be used in predictive modeling to learn the relation between desired feature-vector and labeled classes. When the data set contains arbitrary big number of missed data and/or the amount of data samples is not adequate to the data complexity, it is important to define a strategy that allows to reach highest possible classification accuracy. In this work authors present results on classification-based predictive model's accuracy for three different strategies: input pruning, semi-auto selection of various classification methods, and data volume increase. Authors suggest that a satisfactory level of model's accuracy can be reached when preliminary input pruning is used.
The presented model is connecting fishing data with environmental variables. Even with limited number of samples the model is able to resolve the type of the fish with up to 92% of accuracy.
The results of using various classification methods are shown and suggestions are made towards defining the optimal strategy to build an accurate predictive model, opposed to common trial-and-error method. Different strategies for input pruning that assure information's preservation are described.
Eleni Rozaki
Institute of Technology Tallaght, Ireland
Title: Business analytics applications in budget modelling to improve network performance
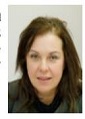
Biography:
Eleni Rozaki obtained an honours degree in economics and earned her M.Sc. degree in Quantitative methods and Informatics from University of Bari Italy. Her PhD degree is in the area of data mining and business analytics in telecommunication networks at Cardiff University, United Kingdom. She has experience as a data analyst in IT and in the telecommunication industry in Ireland. She is currently working as an associate lecturer in Institute of Technology Tallaght, National College of Ireland and Dublin Institute of Technology. Her current research interests include business analytics, predictive modelling, decision support systems and data mining techniques
Abstract:
Efficient and effective network performance monitoring is a vital part of telecommunications networks. Finding and correcting faults, however, can be very costly. In order to address network issues associated with financial losses a model in business analytics for budget planning is presented. The model that is presented is based on previous work of network fault detection, along with cost considerations and customer segmentation. This work focuses on data mining techniques to show the cost probability of the distribution of network alarms based on budget planning classification rules using predictive analytics to determine the minimum bandwidth costs that are possible with network optimisation. The results of the tests performed show that reductions in optimisation costs are possible; some test cases are clustered, of which the results were used to create a performance -based budget model. The results also find out the clients’ demographics, customers’ churn and simultaneously the financial cost of network optimisation in order to review an efficient budget process and improve expenditure prioritisation
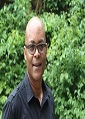
Biography:
Derrick K Rollins is a Professor of Chemical and Biological Engineering and Statistics. He completed his Graduation in Statistics and Chemical Engineering from Ohio State University. He worked at DuPont chemical company for seven years and four months as a Faculty Intern. He received various awards that include: The 2012 Tau Beta Pi McDonald Mentoring Award, the 1996 American Association for the Advancement of Science (AAAS) Mentor Award and National Science Foundation Presidential Faculty Fellows Award. His research areas include “Blood glucose monitoring, modeling and control, and (medical-, bio-, and material-) informatics”.
Abstract:
Advanced statistical methodologies have key roles to contribute in data mining and informatics for large data sets. Over the years, our research has developed a number of statistical techniques exploiting multivariate analysis and methodologies in many applications including bioinformatics, specifically, microarray data sets in a number of applications, medical informatics, including disease diagnosis and discovery, and in material informatics, including the development and evaluation of material properties and testing techniques. In this talk, we present the tools and methodologies that we have developed over the years and discuss their attributes and strengths. The two primary multivariate statistical methodologies that we have exploited have been principal component analysis (PCA) and cluster analysis (CA). This talk will break this technique down for the non-expert and then demonstrate their strengths in handling large data sets to extract critical information that can be exploited in analysis, inference, diagnosis and discovery.
Christophe Jouis
LIP6 (CNRS -UPMC Sorbonne Université), France
Title: Contextual Exploration (EC3): a strategy for the detection, extraction and visualization of target data

Biography:
Witold Dzwinel holds full professor position at the AGH University of Science and Technology, Department of Computer Science in Krakow. His research activities focuses on computermodeling and simulation by using discrete particles. Simultaneously, he is doing research in interactive visualization of big data and machine learning algorithms. Professor Dzwinel is the author and co-author of about 190 papers in computational science, computational intelligence and physics.
Abstract:
EC3 is intended to extract relevant information from large heterogeneous and multilingual text data, in particular in WEB 3.0. The project is based on an original method: contextual exploration. EC3 does not need syntactic analysis, statistical analysis or a "general" ontology. EC3 uses only small ontologies called "linguistic ontologies" that express the linguistic knowledge of a user who must concentrate on the relevant information from one point of view. This is why EC3 works very quickly on large corpus, whose components can be both whole books as well as "short texts": SMS to books. At the output, EC3 offers a visual representation of information using an original approach: the "Memory Islands".EC3 is implanted in the ACASA / LIP6 team. EC3 is tested on the large digitized corpus provided by the Labex OBVIL «Observatoire de la Vie Littéraire», in partnership with the Bibliothèque Nationale de France (http://obvil.paris-sorbonne.fr/). OBVIL intends to develop all the resources offered by digitization and computer applications to examine French literature from the sixteenth to the twentieth century, English and American literature, Italian literature, Spanish literature, in its most traditional formats and media Or the most innovative.
Giovanni Rossi
University of Bologna, Italy
Title: Objective function-based clustering via near-Boolean optimization
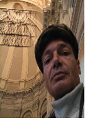
Biography:
Giovanni Rossi completed his PhD in Economics and joined Computer Science department at University of Bologna. His research interests include “Discrete applied mathematics, combinatorial geometries, lattice and pseudo-Boolean functions, network community structure and graph clustering”.
Abstract:
Objective function-based clustering is here looked as a maximum-weight set partitioning combinatorial optimization problem, with the instance given by a pseudo-Boolean (set) function assigning real-valued cluster scores (or costs, in case of minimization) to data subsets, while on every partition of data the global objective function takes the value given by the sum over clusters (or blocks) of their individual score. The instance may thus maximally consist of 2n reals, where n is the number of data, although in most cases the scores of singletons and pairs also fully determine the scores of larger clusters, in which the pseudo- Boolean function is quadratic. This work proposes to quantify the cluster score of fuzzy data subsets by means of the polynomial MLE (multi-linear extension) of pseudo-Boolean functions, thereby translating the original discrete optimization problem into a continuous framework. After analyzing in these terms, the modularity maximization problem, two further examples of quadratic cluster score functions for graph clustering are proposed, while also analyzing greedy (local and global) search strategies.
Abdul Basit
State Bank of Pakistan, Pakistan
Title: Data mining via entropy: Application on trade database
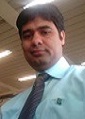
Biography:
Abdul Basit has completed his MS in Social Sciences at SZABIST, Pakistan. Currently, he is the PhD research scholar in the discipline of Statistics at National College of Business Administration & Economics Lahore, Pakistan. He is an Assistant Director of Statistics & DWH Department of State Bank of Pakistan. He has published four research papers in the journals and many articles were presented in national and international conferences.
Abstract:
Entropy is a mathematical tool to gather maximum information about understanding distribution, systems, surveys and databases. We are introducing entropy as a tool of data mining which provides the maximum information about the trade behavior in different regions. In this study, we also derived a new entropy measure for data mining. This study will lead us to explore the new avenues of business and investment in Pakistan. China is the biggest player of global trade from Asian region. To expand the scope of competitiveness, China is continuously investing in different projects around the world. The China–Pakistan Economic Corridor (CPEC) is one of the major projects. The corridor is considered to be an extension of China's economic ambition One Belt-One Road initiative (OBOR). In future China wants to expand her trade with the world using the CPEC to enhance the scope of competitiveness. Pakistan also believes in open trade and continuously trying to enhance trade with the world. To attain maximum advantage of CPEC, Pakistan needs to explore the opportunities for the investors and business communities. In this study, we will develop linkages between the trends of our industries, commodities and their future demand in different regions.
- Young Researchers Forum
Session Introduction
Kruy Seng
Lingnan University, Hong Kong
Title: Cost-sensitive deep neural networks to address class imbalanced problem
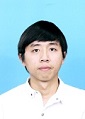
Biography:
Kruy Seng is currently an MPhil student in Department of Computing and Decision Sciences at Lingnan University. His research interests include Data Mining in Business Application and Machine Learning.
Abstract:
Class imbalance is one of the common issues in data mining. Most data in real-life applications are imbalanced, that is, the number of examples belonged to a class is significantly greater than those of the others. Abnormal behaviors or rare events are the main reasons that cause the distribution of classes to be imbalanced. The scarcity of minority class examples leads the standard classifiers to be more focused on the majority class examples while ignores the other. As a result, classification becomes more challenging. A number of classification algorithms were proposed in the past decades; however, they are accuracy-oriented and unable to address class imbalanced problem. Much research has been conducted to address this problem. However, it is still an intensive research topic. In this work, we propose an approach called class-based cost-sensitive deep neural networks to perform classification on imbalanced data. The costs of misclassification of each type of errors are treated differently and are incorporated into the training process of deep neural networks. We also generalize the method by reducing the effort of hyper parameter selection by adapting evolutionary algorithm search for optimal cost vector setting and network structure. Finally, experiments will be conducted to analyze the performance and compare with other existing methods.
Prajakta Diwanji
FHNW University of Applied Sciences and Arts Northwestern
Title: Data driven intelligent analytics in education domain
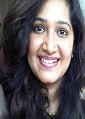
Biography:
Prajakta Diwanji is working as a Researcher in Information Systems at University of Applied Sciences and Arts, Northwestern Switzerland (FHNW). She is a first-year Doctoral student at University of Camerino, Italy. Her research interest is in the area of intelligent data analytics in education domain. She has completed her Master’s degree in Business Information Systems from FHNW, Switzerland, and Masters in Computer Science at University of Pune, India. She has more than seven years of work experience in IT industry where she has taken up several challenging roles. During this tenure, she has worked with international companies like Roche Pharma, Switzerland and IBM, India etc.
Abstract:
In recent times, there is a steady growth in student’s personal as well as academic data in the education field. Many universities and institutes have adopted information systems like virtual learning environments, learning management systems and social networks that collect student’s digital footprints. This data is both large in volume and diversity. Learning analytics offers tools to facilitate the understanding of different parameters related to student’s engagement/motivation, learning behavior, performance, teaching content and learning environment. Such information could help teachers better prepare for the classroom sessions and to deliver personalized or adaptive learning experiences. This, in turn, could enhance student performance. The current literature research states that there is a shift of focus from classroom based learning to a more anytime, anywhere learning; as well as from a teacher as a sole knowledge contributor to agent or learner as a contributor towards learning. The use of intelligent digital tutors/chatbots has taken the learning process to a new level of student engagement, interaction, and learning. Such intelligent data analysis tools/systems make use of data analysis techniques like machine learning, natural language processing etc. along with artificial/cognitive intelligence techniques. The research work identifies the current challenges faced by universities in learning/teaching processes in a real world context and tries to address these problems using data driven intelligent analysis methods. The main goal would be to focus on preparing students as well as lecturers effectively for the classroom lectures; to understand learning needs of students beforehand; to address those needs proactively in a timely manner.
SASA Yuko
University Grenoble Alps, France
Title: The Domus-LIG Experimental Living-Lab methodology and the EmOz platform: overview of spontaneous and ecological corpora - from Small Smart Data to Big Data
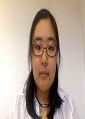
Biography:
Yuko SASA is a young researcher in the field of social robotics, finishing a PhD the LIG - computer science lab and financed by the Labex Persyval-lab. She completed a computational linguistics Master in 2012, and a Gerontechnology Master in 2013, at Grenoble Alps University. Her supervisors are V. Aubergé (LIG), G. Feng (Gipsa-Lab) and Y. Sagisaka (Waseda University). She is enrolled in several academic committees and was selected for international research programs as the French-American Doctoral Exchange Seminar on Cyber-Physical Systems (FadEx - French Embassy), or the ROW (Research Opportunities Week - Technical University of Munich).
Abstract:
The availability of real-life data through the IoT and ICT technologies, completed by the augmenting computers' calculability have developed the paradigm of Big Data, to process an enormous amount of information to automate various system as speech technologies and robotics. The motivation resided on the "intelligence" of data to handle the naturalness and variability of the human behaviors. The actual machine learning techniques, as DNN, let the computer mathematics to approximate and to generalize the cognitive processes more than modelizing them. The quantity of data aim is then to cover the knowledge, which is poorly explicit. They maybe rely on too many implicit mechanisms locked up into black boxes. The Domus-LIG Experimental Living-Lab methodology and the EmOz platform, a wizard of oz tools interfaced with a robot, both developed to induce, observe and collect spontaneous and ecological interactional data of human-robot communication, particularly with socio-affective values. The robot is thus a measuring instrument of the human multimodal speech features' effects, strongly hypothesized. This methodology works on agile loops of processing to control the data contents and format them in short and rapid processes. This evolving corpus is the basis for an iterative machine learning, dedicated to automatic recognition systems. Different studies leading to the EEE (EmOz Elderly Expressions) or the GEE (Gesture EmOz Expressions) corpora this approach. This bootstrap is an invitation to discuss the possible mechanisms to move from Small Smart Data to relevant Big Data.
- Young Researchers Forum
Session Introduction
Febriana Misdianti
University of Manchester School of Computer Science, UK
Title: Implementation and performance comparison of Wilson, holdout, and Multiedit algorithm for edited k-nearest neighbor
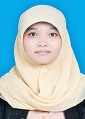
Biography:
Febriana Misdianti is a Post-graduate student at University of Manchester. She has completed her Bachelor degree of Computer Science at Universitas Indonesia and has two years of working experience in startup companies in Jakarta and Singapore. She has been winning a lot of competitions related to computer science and has published a paper about data security in a reputable journal.
Abstract:
K-nearest neighbor (k-NN) classifier is widely used for classifying data in various segments. However, k-NN classifier has high computational cost because it uses linear search through all training data. In naïve implementation, k-NN will go through all training set i to compute its distance d from input data (O(nd)). Then, it loops again for all training set n to find k smallest results (O(nk)). So, the overall time complexity for k-NN is O(nd+nk). Thus, it is not suitable to classify multidimensional data with a huge number of training set. Meanwhile, k-NN needs a large number of samples in order to work well. There have been several ideas proposed to increase the time performance of k-NN in predicting a test data. One of the popular ideas is by reducing the number of TS in a model so that it would cut the testing time as the number of data that need to be explored becomes smaller. The aim of this experiment is to implement k-NN editing algorithms that cut the number of training data so that it become faster in predicting an input. This experiment implements three editing algorithms, namely, Wilson’s editing, holdout editing, and Multiedit algorithm, and also compare the performance of them.
Sofie De Cnudde
Antwerp University, Belgium
Title: Deep learning classification for large behavioral data
Biography:
Sofie De Cnudde is currently finishing her PhD at the University of Antwerp in Belgium. Her PhD analyses and compares the performance of wide and deep classification techniques on large and sparse human behavioral data. She has given tutorials on the use of deep learning for large-scale classification and has currently finished a research stay at an e-commerce company in London. Her work has been published in Expert Systems with Applications, Decision Support Systems and has been presented at conferences such as IFORS.
Abstract:
Deep learning has demonstrated significant performance improvements over traditional state-of-the-art classification techniques in knowledge discovery fields such as computer vision and natural language processing. Moreover, the representation-learning nature of the deep learning architecture enables intuitive interpretation of these complex models. The predictive analysis of very high-dimensional human behavioral data (originating from contexts such as e-commerce) could highly benefit from a complex classification model on the one hand and from an intuitive insight in the many fine-grained features on the other hand. The research question we investigate is whether and how deep learning classification can extend its superior results to this omnipresent type of big data. We present the following three contributions. First and foremost, the results of applying deep learning on large, sparse behavioral data sets demonstrate as good as or better results compared to shallow classifiers. We find significant performance improvements over linear support vector machines, logistic regression with stochastic gradient descent and a relational classifier. Moreover, we shed light on hyper-parameter values to facilitate the adoption of deep learning techniques in practice. The results demonstrate that an unsupervised pre-training step does not improve classification performance and that a tanh non-linearity achieves the best predictive performance. Lastly, we entangle the meaning of the neurons in a manner that is intuitive for researchers and practitioners, and show that the separate neurons identify more nuances in the many fine-grained features compared to the shallow classifiers.
- Video Presentation
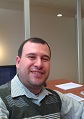
Biography:
Joseph Bonello is an Assistant Lecturer at University of Malta. He has worked on various EU related projects. He worked on various IT projects for the Department of Customs. He has managed the development of the IRRIIS project for a local member of the consortium. He has experience in developing commercial applications including work for local banking firms and government. He completed his BSc (Hons) in IT in 2002 at University of Malta and Master’s degree at University of Malta on Automated Detection and Optimization of NP-Hard Scheduling Problems in Manufacturing.
Abstract:
Many IT organizations today spend more than 40% of the project’s budget on software testing (Capgemini, 2015; Tata, 2015). One way of making the testing process easier is to automatically generate synthetic data that would be similar to the data inputted in the real environment. The benefits offered by an automated process are invaluable since real datasets can be hard to obtain due to data sensitivity and confidentiality issues. Automated Dataset Generator (ADaGe) enables the end-user to define and tweak a dataset definition through an interface in a way that satisfies the system’s requirements. Our tool enables the automatic generation of small to large amount of synthesized data that can be outputted to a file or streamed to a real-time processing system.
Jose Manuel Lopez-Guede
University of the Basque Country (UPV/EHU), Spain
Title: Data Mining applied to Robotics
Biography:
Jose Manuel Lopez-Guede received the Ph.D. degree in Computer Sciences from Basque Country University. He got 3 investigation grants and worked in a company 4 years. Since 2004 he worked as full time Lecturer and since 2012 as assoc. prof. He has been involved in 24 competitive projects and published more than 100 papers, 25 on Educational Innovation and the remaining in specific research areas. He has 20 ISI JCR publications, more than 15 other journals and more than 40 conferences. He has belonged to more than 10 organizing committees of international conferences and to more than 15 scientific committees.
Abstract:
One of the key activities of Data Mining is to discover and make clear hidden relations and working rules in complex systems. Robotics is a complex scope in which first prinicples approaches have been used to solve straight problems, but that approach is not enought to deal with complex problems, where more intelligence-based approaches are needed. Data Mining can be used for autonomous learning of control algorithms for Linked Multicomponent Robotic Systems (L-MCRS), because it is an open research field. Single Robot Hose Transport (SRHT) is a limit case of this kind of systems, when one robot moves the tip of a hose to a desired position, while the other hose extreme is attached to a source position. Reinforcement Learning (RL) algorithms have been applied to learn autonomously the robot control in SRHT, specifically Q-Learning and TRQ-Learning have been applied with success. However storing the state-action value functional information in tabular form produces large and intractable data structures, and using the Data Mining approach the problem can be addressed by discivering and learning the state-action values of Q-table by Extreme Learning Machines (ELM), obtaining a data reduction because the number of ELM parameters is much less than the Q-table's size. Moreover, ELM implements a continuous map which can produce compact representations of the Q-table, and generalizations to increased space resolution and unknown situations.
- Poster Presentation