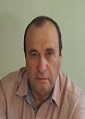
Boris Mirkin
Higher School of Economics,Russia
Title: A Complementary Square-Error Clustering Criterion and Initialization of K-Means
Biography
Biography: Boris Mirkin
Abstract
Clustering is a set of major data analysis techniques. The square-error clustering criterion underlies most popular clustering methods including k-means partitioning and Ward agglomeration. For the k-means, the square-error criterion to be minimized is the sum of squared Euclidean distances from all the objects to their respective cluster centers/means, W(S,c), where S is the sought partition of the set of objects and c is the set of within-cluster means. The method’s popularity stems from the simplicity of computation and interpretation. Yet there is a catch: the user is to specify both the number of clusters and the initial locations of cluster centers, which can be of an issue sometimes. To tackle the problem, the current author proposes using the complementary criterion. It is not difficult to prove that there is a complementary criterion, B(S,c), to be maximized, such that W(S,c)+B(S,c)=T where T is the data scatter. The complementary criterion B(S,c) is the sum of individual cluster contributions, equal each to the product of the cluster’s cardinality and the squared Euclidean distance from the cluster’s center to 0. Therefore, the complementary criterion leads to a set of anomalous clusters, which can be found either one-by-one or in parallel. Our experiments show that methods emerging in this perspective are competitive, and frequently superior, to other initialization methods.
Speaker Presentations
Speaker PPTs Click Here