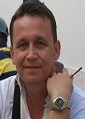
Witold Dzwinel
AGH University of Science and Technology, Poland
Title: A novel linear-time and memory saving approach for visual exploratory data analysis: data embedding and graph visualization
Biography
Biography: Witold Dzwinel
Abstract
Data embedding (DE) and graph visualization (GV) methods are very congruent tools used in exploratory data analysis for visualization of complex data such as high-dimensional data and complex networks, respectively. However, high computational complexity and memory loads of existing DE and GV algorithms (based on t-SNE concept from one hand, and force-directed methods from the other), considerably hinders visualization of truly large and big data consisting of as many as M~106+ data objects and N~103+ dimensions. In this paper, we demonstrate the high computational efficiency and robustness of our approach to data embedding and interactive data visualization. We show that by employing only a small fraction of distances between data objects, one can obtain very satisfactory reconstruction of the topology of N-D data in 2D in a linear-time O (M). The IVHD (Interactive Visualization of High-Dimensional Data) method quickly and properly reconstructs the N-D data topology in a fraction of computational time required for the state-of-art DE methods such as bh-SNE and all its clones. Our method can be used for both metric and non-metric (e.g. large graphs) data visualization. Moreover, we demonstrate that even poor approximations of the nearest neighbor (NN) graph, representing high-dimensional data, can yield acceptable data embedding. Furthermore, some incorrectness in the nearest neighbor list can often be useful to improve the quality of data visualization. This robustness of IVHD, together with its high memory and time efficiencies, meets perfectly the requirements of big and distributed data visualization, when finding the accurate nearest neighbor list represents a great computational challenge.
Speaker Presentations
Speaker PPTs Click Here