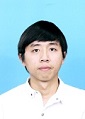
Kruy Seng
Lingnan University, Hong Kong
Title: Cost-sensitive deep neural networks to address class imbalanced problem
Biography
Biography: Kruy Seng
Abstract
Class imbalance is one of the common issues in data mining. Most data in real-life applications are imbalanced, that is, the number of examples belonged to a class is significantly greater than those of the others. Abnormal behaviors or rare events are the main reasons that cause the distribution of classes to be imbalanced. The scarcity of minority class examples leads the standard classifiers to be more focused on the majority class examples while ignores the other. As a result, classification becomes more challenging. A number of classification algorithms were proposed in the past decades; however, they are accuracy-oriented and unable to address class imbalanced problem. Much research has been conducted to address this problem. However, it is still an intensive research topic. In this work, we propose an approach called class-based cost-sensitive deep neural networks to perform classification on imbalanced data. The costs of misclassification of each type of errors are treated differently and are incorporated into the training process of deep neural networks. We also generalize the method by reducing the effort of hyper parameter selection by adapting evolutionary algorithm search for optimal cost vector setting and network structure. Finally, experiments will be conducted to analyze the performance and compare with other existing methods.