Day 2 :
- Data Mining Applications
Session Introduction
E. Cabral Balreira
Trinity University, USA.
Title: Using an Oracle to improve the Quality of Predictions.
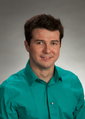
Biography:
E. Cabral Balreira is an associate professor of mathematics at Trinity University in San Antonio, Texas. His main area of research is differential topology with applications to global invertibility and discrete dynamics. Motivated by his interest in mathematics and sports, Balreira and his colleagues at Trinity developed a mathematical method, the Oracle, to rank sports teams and predict tournament outcomes. Balreira received his Ph.D. from the University of Notre Dame in 2006.
Abstract:
There are many different approaches to rank teams in the NFL and NBA. One is able to make predictions of game outcomes and decide postseason odds. In this project, we are in interested in quantifying the accuracy and improving the quality of the predictions given by a particular ranking model. We introduce a method to address the over-confidence of game predictions that increases the quality of predictions when assessed by standard scoring metrics. In addition, we develope a method to better include homefield advantage in a ranking method. We evaluate our predictions in the past 15 years of NFL and NBA and show that our newly developed ranking method called the Oracle consistently outperformes currently available computer models in accuracy and quality of predictions.
Saima Kashif
NED University of Engineering and Technology, Pakistan
Title: Virtual Screening of Natural Compounds that can Inhibit HCV
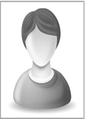
Biography:
Ms. Saima Kashif has completed Masters in Biomedical Engineering with dissertation with 3.83CGPA from NED University of Engineering and Technology Karachi Pakistan. Currently she is working as a Lecturer and research supervisor at Department of Biomedical Engineering, NED University of Engineering and Technology Karachi, Pakistan.
Abstract:
Hepatitis C virus (HCV) is highly prevalent in Pakistan, and it’s infection can lead to chronic liver disease and hepatocellular carcinoma. The currently available treatment for treating HCV infection is not effective in all patients, has adverse side effects, and is not easily affordable. The aim of this study was to explore for the natural compounds that can inhibit the replication of Hepatitis C virus (HCV). Fluoroquinolones are the chemicals known as potent- active compounds that inhibit the replication of HCV genome by targeting its helicase protein NS3. Using 40 fluoroquinolones as reference molecules, a data set of 4000 natural products was screened that bore structural similarities with fluoroquinolone. From this data set, Random Forest classifier was used to predict active natural compounds that may have an inhibitory effect against HCV NS3 activity. This Random Forest classifier builds a set of decision trees by using a set of 0D, 1D, 2D, 3D and others, total 2080 molecular descriptors, of the two data sets i.e., the training and testing set. Compounds with RF score >0.5 are classified as an active compound against HCV. Using this approach, out of 4000 test molecules, 147 molecules were predicted to be active against HCV NS3 helicase. These predicted active compounds can be analyzed further using in silico and in vitro experimental models to discover a effective drug against HCV. The above-described approach is useful in discovering new, more portent and affordable drugs for treating HCV infection.
Sadaf Naeem
University of Karachi, Pakistan.
Title: Virtual Screening of Phytochemical Constituents from Traditional Indonesian Herbs for Inhibitors of Aldose Reductase
Biography:
Sadaf Naeem is currently working in Biochemistry department in University of Karachi, Pakistan
Abstract:
Data on phytochemical constituents of herbs commonly used in traditional Indonesian medicine have been compiled as a database using ChemDBSoft software. This database (the Indonesian Herbal constituents database, IHD) contains details on 1,242 compounds found in 33 different plants. For each entry, the IHD gives details of chemical structure, trivial and systematic name, CAS registry number, pharmacology (where known), toxicology (LD50), botanical species, the part(s) of the plant(s) where the compounds are found, typical dosage(s) and reference(s). A second database has been also been compiled for plant-derived compounds with known activity against the enzyme, aldose reductase (AR). This database (the aldose reductase inhibitors database, ARID) contains the same details as the IHD, and currently comprises information on 112 different AR inhibitors. In the search for novel leads active against AR to provide for new forms of symptomatic relief in diabetic patients – virtual screening of all compounds in the IHD has been performed using (a) random forest (RF) modelling, and (b) molecular docking. For the RF modelling, 3 sets of chemical descriptors – Constitutional, RDF and 3DMoRSE (computed using the DRAGON software) were employed to classify all compounds in the combined ARID and IHD databases as either active or inactive as AR inhibitors. The resulting RF models (which give misclassification rates of ~10%) were used to identify putative new AR inhibitors in the IHD, with such compounds being identified as those giving a mean RF score > 0.5. Virtual screening of the IHD was also performed using the docking software, Molegro Virtual Dokcer (MVD), In the docking studies reported here, carboxyl-containing IHD compounds were docked in to the active site of the 11 crystal structures of AR bound to different carboxyl containing ligands. Calculation of the averages of these 11 MVD re-rank scores was carried out as a means to identify anomalous docking results. In-vitro assays were subsequently performed to determine the inhibitory activity against human recombinant AR for four of the compounds obtained as hits in RF in-silico screenings and for a further four compounds obtained as hits in the docking studies. All four of the RF and docking hits were (as predicted) active as AR inhibitors with IC50s in the micromolar range.
Biography:
He holds PhD - Iraq - Tikrit University - Faculty of Education - Department of Geography - specialty maps and geographic information systems ,A member of the Iraqi Geographic Society 2011 , Director of the Center for Idrisi geographic techniques - Fallujah, Iraq Member of the Union of Iraqi Writers -2004, Global certification test certificate Ic3 Computers and Information Technology. Research published in the Iraqi magazines (4) in Arabic magazines (3) in international journals (4) influential factor in international journals (Impact Factor) number (2) the number of published books (2) the number of books in print (3) BOOKS (2) Presenting
Abstract:
This paper presents how to prepare an advanced database and employ Web maps available on the Internet through the application of (ArcGIS On Line) to the city of Kirkuk, a case study. The design of effective maps on the website allows display data effectively so as to encourage the user to make a decision. Use this application to the preparation of land use maps (Health -Education - Recreational - Other - Land space). The search area, the adoption of data open source, was painted spatial layers to land use on an individual basis through the use of spatial symbols available in the application interface, and then assembled in the map final collected, to be beyond the possibility of participation through the media and other programs such as (Google Earth) can made available to decision-makers. The research aims to use Web maps available on the Internet data through the application of (ArcGis On Line) and applied to the city of Kirkuk - the military district case study, and perhaps one of the most sophisticated tools are interactive maps of the data effective Aljgraveh.valtsamam maps on the website allows viewing data effectively so as to encourage the user to make a decision. Follow the search according to the style of practical work through the use of geographic and spatial data available on the Internet to prepare the land use map in the search area, and drawing classes and coded down to the interactive map can be shared and disseminated.
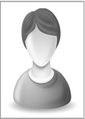
Biography:
Archana Shukla has done her Ph.D in computer science department from Motial Nehru National Institute of technology Allahabad. She is currently working as a Visiting Faculty in computer science department.
Abstract:
- Data Mining Tools and Software
Session Introduction
Ahmed N. AL-Masri
American University in the Emirates, UAE
Title: Implementing Machine Learning for Big Data Analytics, Challenges and Solutions
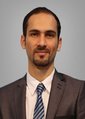
Biography:
Dr. Ahmed AL-Masri received his Ph.D degree in the field of Artificial Intelligence application from University Putra Malaysia. He has more than 6 years’ experience in teaching, programming and research. He involved in many project used artificial neural network system such as forecasting, online monitoring, smart grid, security assessment in electrical power system and dynamic system stability. He is acting as a reviewer for various International and National Journals, and Member of the Institute of Electrical and Electronic Engineers (IEEE). His professional expertise is in the design, analysis of artificial intelligence system, security assessment, parallel processing, virtualization, cloud computing and system automation.
Abstract:
Big Data analytics is one of the great trials in Learning Machine algorithms, as most of real life applications include a massive information or big data knowledge base. On the other hand, the artificial intelligent system with data knowledge base should be able to compute the result in very accurate and fast manner. This paper focused on the challenges and solutions of using Learning Machine with Big Data. Data processing is a mandatory step to transform Big Data which is unstructured into meaningful and optimized data set in any LM module. However, it is necessary to deploy an optimized data set to support the distributed processing and real-time application. This work also reviewed the current used technologies on the Big Data analysis and LM computation. The revision emphasizes on the viability of using different solution for a certain applications could increase the performance of LM. The new development especially in cloud computing and data transaction speed gives more advantages to the practical use of artificial intelligence applications.
Hooman Fallah
Islamic Azad University, Iran
Title: Field scale simulation study of miscible water alternating co2 injection process in fractured reservoirs
Biography:
Abstract:
Vast amounts of world oil reservoirs are in natural fractured reservoirs. There are different methods for increasing recovery from fractured reservoirs. Miscible injection of water alternating CO2 is a good choice among this methods. In this method, water and CO2 slugs are injected alternativelyin reservoir as miscible agent into reservoir. This paper studies water injection scenario and miscible injection of water and CO2 in a two dimensional, inhomogeneous fractured reservoir. The results show that miscible water alternating CO2 gas injection leads to 3.95% increase in final oil recovery and total water production decrease of 3.89% comparing to water injection scenario.
- Artificial Intelligence
- Big Data Applications
Location: USA
Session Introduction
Alex V. Vasenkov
Multi Scale Solutions, USA
Title: Big Data for Research and Development
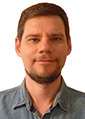
Biography:
Dr. Vasenkov has received a Ph. D. degree in Physics and Mathematics from the Russian Academy of Science in 1996. Dr. Vasenkov’s research was funded through peer-reviewed grants and contracts from major federal agencies including the DOE, NSF, and the DoD and private companies such as Samsung Advanced Institute of Technology. In 2013, he co-founded a small-business company Multi Scale Solutions Inc. focusing on the development and commercialization of scientific business intelligence software. Dr. Vasenkov is executive editor of Journal of Nanomedicine & Nanotechnology. He authored/co-authored over 30 publications in peer-reviewed journals, 1 book chapter, and over 50 presentations at leading scientific conferences.
Abstract:
This talk will focus on Big Data for Research and Development (R&D). There are several definitions of Big Data which create confusion about this subject. There is even more confusion about synthetic big data that can be defined as a collection of research articles, Ph. D. theses, patents, test reports and product description reports. Such data have emerging attributes like high volume, high velocity, high variety, and veracity that make an analysis of synthetic data difficult. There is an emergent need for a framework that can synergistically integrate search or information retrieval (IR) with information extraction (IE). Traditional IR-based text searching can be used for a quick exploration of large collections of synthetic data. However, this approach is incapable of finding specific R&D concepts in such collections and establishing connections between these concepts. Also, the IR models lack an ability to learn concepts and relationships between the concepts. In contrast, the IE models are too specific and typically require customization for a domain of interest. A novel framework will be presented and its feasibility to mine synthetic data will be shown. It was found possible to partially or fully automate analysis of synthetic data to find labeled information and connecting concepts. The present framework can help individuals to identify non-obvious solutions to R&D problems, to serve as an input for innovation, or to categorize prior art relevant to a technological concept or a patent application in question.
Pengchu Zhang
Sandia National Laboratories, USA
Title: Enhancement of Enterprise Search with Neural Language Model
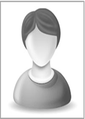
Biography:
Pengchu Zhang has more then ten years experiences in computer modeling/simulation, machine learning, data mining and unstructured data analysis at Sandia National Laboratories. His recent interesting is to develop and apply technologies of Deep Learning in enterprise knowledge sharing and management.
Abstract:
A significant problem that reduces the effectiveness of enterprise search is query terms that do not exist in the enterprise data. Consequently, enterprise search generates no results or the answers match the exact query terms and do not take into account related terms. This results in a high rate of false positives in terms of information relevance. Recent developments in neural language model (NLM), specifically, the word2vec model initiated by Google researchers has drawn a great deal of attention in last two years. This model uses multiple layers of neural networks to represent words into vector spaces. The vector representation of words carries both semantic as well syntactic meanings. Terms with the semantic similarities are close together in the vector space as measured by their Euclidean distances. Enterprise search may utilize the “contextual†relationships between words to intelligently increase the breath and quality of search results. Application of the NML in our enterprise search promises to significantly improve the findability and relevance of returned information. We expand the query term(s) into a set of related terms using the trained term vectors based on corporate data repositories as well as well as making use of Wikipedia. The expanded set of terms is used to search the indexed enterprise data. The most relevant data rises in ranking including documents which may not contain the original query terms. In this presentation, we will also discuss the potential and limitations of applying NLM in search and other aspects of enterprise knowledge management.
Ibrahim Abaker Targio Hashem
University of Malaya, Malaysia
Title: Schedule Optimization for Big Data Processing on Cloud
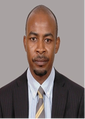
Biography:
Ibrahim Abaker Targio Hashem is currently a Ph.D. degree candidate at the Department of Compute Systems UM; He has been working on big data since 2013, his article on big data becomes top most downloaded in 2014 Information System journal 2013 elsevier. He has experienced on configuring Hadoop MapReduce in Multi-node cluster. His main research interests include big data, cloud computing, distributed computing, and network.
Abstract:
Over the past few years, the continuous increase in computational capacity has produced an overwhelming flow of data or big data, which exceeds the capabilities of conventional processing tools. Big data offer a new era in data exploration and utilization. The major enabler for underlying many big data platforms is certainly the MapReduce computational paradigm. MapReduce is recognized as a popular programming model for the distributed and scalable processing of big data and is increasingly being used in different applications mostly because of its important features that include scalability, flexibility, ease of programming, and fault-tolerance. Scheduling tasks in MapReduce across multiple nodes have shown to be multi-objective optimization problem. The problem is even more complex by using virtualized clusters in a cloud computing to execute a large number of tasks. The complexity lies in achieving multiple objectives that may be of conflicting nature. For instance, scheduled tasks may require to make several tradeoffs between the job performance, data locality, fairness, resource utilization, network congestion and reliability. These conflicting requirements and goals are challenging to optimize due to the difficulty of predicting a new incoming job’s behavior and its completion time. To address this complication, we introduce a multi-objective approach using genetic algorithms. The goal is to minimize two objectives: execution time and budget of each node executing the task in the cloud. The contribution of this research is to propose a novel adaptive model to communicate with the task scheduler of resource management. The proposed model periodically queries for resource consumption data and uses to calculate how the resources should be allocated to each task. It passes the information to the task scheduler by adjusting task assignments to task nodes accordingly. The model evaluation is realized in scheduling load simulator. PingER is the Internet End-to-End performance measurement, was chosen for performance analysis of the model. We believe this proposed solution is timely and innovative as it provides a robust resource management where users can perform better scheduling for big data processing in a seamless manner.
David Sung
KyungHee University School of Management Graduate School, South Korea
Title: The Effects of Google Trends on Tourism Industry in South Korea
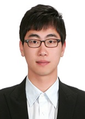
Biography:
David Sung is a graduate student in the Master Program for Information Management at Kyunghee University, Seoul, Korea. He has a B.A. in Economics and applied mathematics from Konkuk University. Also, Sang-Hyun Park is a graduate student in the Master Program for Information Management at Kyunghee University, Seoul, Korea. And he has a B.A department of applied organic materials engineering from Inha University.
Abstract:
This study deals with the effects of an online search engine on the level of tourism through analyzing any change in the amount of searched information. The paper has the purpose to improve the forecast of tourism industry into South Korea by utilizing of Google Trends data which provide the data is provided in a relative query and time series format of data. This particular work attempts to analyze Google Trends based on with 3 specific keywords, “hotels, flights and tour†that are searched by potential tourists around the world from the world to South Korea. We conduct this study through a series of multiple regression models with one- month time-lag in order to forecast for easily forecasting performance. Accordingly, the findings suggest that Google Trends can be a good source of estimating tourism industry. Therefore, the business strategy makers in tourism industry related of South Korea can easily utilize of the Google Trends data for their future decisions. This work was supported by the National Research Foundation of Korea Grant funded by the Korean Government (NRF-20141823)
- Artificial Intelligence
Location: USA
Session Introduction
Mohamed M. Mostafa
Gulf University for Science and Technology, Kuwait
Title: Oil price forecasting using gene expression programming and artificial neural networks
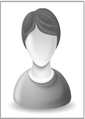
Biography:
Mohamed M. Mostafa has received a PhD in Business from the University of Manchester, UK. He has also earned a MS in Applied Statistics from the University of Northern Colorado, USA, a MA in Social Science Data Analysis from Essex University, UK, a MSc in Functional Neuroimaging from Brunel University, UK, a MBA and a BSc at Port Said University, Egypt. He has published over 60 research papers in several leading academic peer reviewed journals
Abstract:
Mohamed M. Mostafa has received a PhD in Business from the University of Manchester, UK. He has also earned a MS in Applied Statistics from the University of Northern Colorado, USA, a MA in Social Science Data Analysis from Essex University, UK, a MSc in Functional Neuroimaging from Brunel University, UK, a MBA and a BSc at Port Said University, Egypt. He has published over 60 research papers in several leading academic peer reviewed journals
SookYoung Son
Hyundai Heavy Industries, South Korea
Title: A case study on the application of process mining techniques in offshore plant construction process analysis
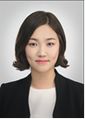
Biography:
SookYoung Son is staff of Hyundai Heavy Indsutreis, the world’s largest shipbuilding company. She has completed her MS in Management Engineering at the Ulsan National Institute of Science and Tehcnology (UNIST). Her research interests include process mining, manufacturing process analysis, data mining, and supply chain management.
Abstract:
Construction of an offshore plant is a huge project involves many activities and resources. Furthermore, multiple projects are usually carried out simultaneously. For this reason, it is difficult to manage the offshore plant manufacturing processes. To improve current processes, it is important to analyze and find problems while the project is ongoing. That analysis usually requires a few months to perform. To shorten the analysis period, this study proposes a method of analyzing offshore plant manufacturing processes using process mining. Process mining is a technique that derives useful information related to processes from the event logs in information systems. It generates a process model from event logs and measures performance of processes, tasks, and resources. In the proposed method, a process model can be generated from the data of several simutaneous projects. The generated process model is used to analyze the overall processes in a company. The generated process model then can be compared to the planned process model to find delays. Moreover, workload analysis can be conducted in terms of resources capacity. Actual workloads for each department can be calculated and compared to its capacity. To verify the proposed process mining method, a case study is conducted. The proposed method was applied to analyze the offshore plant construction process in a heavy industry company in Korea.
Ch.Mallikarjuna Rao
Gokaraju Rangaraju Institute of Engineering and Technology , India ,
Title: Segmentation Based Clustering for Knowledge Discovery from Spatial Data
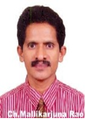
Biography:
Ch.Mallikarjuna Rao Received his B.Tech degree in computer Science and engineering from Dr.Baba sahib Ambedkar Marathwada University, Aurangabad, Maharastra.India in 1998, and M.Tech Degree in Computer Science and Engineering from J.N.T.U Anantapur ,Andhrapradesh,India in 2007. He is currently pursuing his Ph.D degree from JNTU Ananthapur University, Andhra Pradesh. Currently he is working as Associate Professor in the department of Computer Science and Engineering of Gokaraju Rangaraju Institute of Engineering and Technology, Hyderabad,Telangana stat, India. His research interest includes Data bases and data mining.
Abstract:
Global Positioning Systems (GPS) and other data acquisition systems have been collecting huge amount of geographical data that is growing in exponential growth. Mining such data can extract unknown and latent information from spatial datasets that are characterized by complexity, dimensionality and large size. However, it is challenging to do so. Towards this end geographical knowledge discovery through spatial data mining has emerged as an attractive field that provides methods to leverage useful applications. Remote sensing imagery is the rich source of geographical data. Analyzing such data can provide actionable knowledge for making strategic decisions. In this paper we proposed a methodology that is used to perform clustering on remote sensing images. The data sets are collected through World Wind application provided by NASA. The images are with .TIF extension. The methodology includes feature extraction, training, building classifier and cluster analysis. We built a prototype application that demonstrates the proof of concept. The implementation has taken native method support from Fiji and Weka to realize the proposed methodology. The empirical results revealed that the spatial clustering is made with high accuracy.
Mallikarjuan Rao. G
Gokaraju raju Rangaraju Institute of Engineering and Technology,India
Title: Mining of Facial Features for Gender and Expression Classification
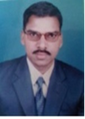
Biography:
Mallikarjuna Rao G. attained B.Tech degree from Nagarjuna University in Electronics and Communication in 1988. He got his first post graduation degree , M.E from Osmanina University in 1992 in Digital Systems. His second post graduate, M.Tech, is obtained from Jawaharalal Nehru Technological university , Hyderabad in Computer Science and Engineering in 2002. He is pursuing P.hD from JNTUH. His research interests are Pattern Recognition , parallel computing and artificial neural networks. Professor Rao has 12 publications in various international journals and conferences. He is established Parallel Computing and Operating Systems Lab at GRIET under MODROB scheme. He proposed salable and portable feature extraction technique Local Active Pixel Pattern ,LAPP.
Abstract:
Facial features if properly analyzed /mined are capable of providing information about the gender classification, expression classification and age approximation. Each one of the task is challenging for the researchers due to the involved computational effort and dynamism associated with environmental conditions of capturing domain. The recent trends in machine based human facial image processing are concerned with the topics about predicting age, classifying gender, race, and expressions from facial images, and so on. However, machine based approaches have not yet captured intelligence of mining process involved in human brain. More over researchers attempted each one as a separate problem and suggested data dependent solutions. Further the algorithms have not exploring available parallel computational power existing machines multi-core machines. Hence, in this paper we would like to propose unified scalable approach based on artificial neural network with a new pre-processing feature extraction technique, local active pixel pattern, LAPP. Intelligence extracted from pixel level mining is induced in the machine so that after training it is capable of classifying the mood of the person. Heterogeneity of excremental data sets have truly demonstrated the significance of our approach. FERET facial database has been used for gender classification , YALE, JAFFE, Face expression and CMU AMP Face Expression Databases have been used for expression classification and FGNET AGE data set has been used for age approximation.Results are encouraging with more the 90% accuracy.
- Data Mining Methods and Algorithms
Session Introduction
Pilar Rey del Castillo
Instituto de Estudios Fiscales, Spain
Title: Big Data for Official Statistics
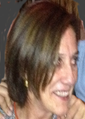
Biography:
Pilar Rey del Castillo is a statistician with 35 years of experience. She got a degree in Mathematics from the Autonomous University of Madrid, a master degree in Time Series from the Bank of Spain and a PhD (Computer Sciences and Artificial Intelligence) from the Technical University of Madrid. Senior Statistician of the Spanish administration, she has performed different functions in the Spanish National Statistical Institute, the Spanish Sociological Research Center, and, currently, the Spanish Fiscal Studies Institute. She also worked as an assistant professor from 1994 to 1998 in the Carlos III University and, from 2011 until 2015, as an expert in Eurostat, European Commission.
Abstract:
The availability of copious data about many human, social and economic phenomena is being nowadays considered as an opportunity for the production of official statistics. National statistical organizations and other institutions are more and more involved in new projects for developing what sometimes is seen as a possible change of paradigm in the way statistical figures are produced. Nevertheless, there are hardly any systems in production using Big Data sources. Arguments of confidentiality, data ownership, representativeness and others make it a difficult task to get results in the short-term. Using Call Detail Records from Ivory Coast as illustration, this paper shows some of the issues that must be dealt with when producing statistical indicators from Big Data sources. A proposal of a specific method to evaluate the quality when using the data to compute figures of daily commutes between home and work is also presented.
Abdulmohsen Algarni
King Khalid University, Saudi Arabia
Title: Selecting Training Documents for Better Learning
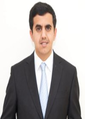
Biography:
Abdulmohsen Algarni received the PhD degrees in the Faculty of Information Technology at Queensland University of Technology, Brisbane, Australia in 2011. He is currently an assistant professor in the Department of Computer Science, king Khalid University. His research interests include web intelligence, data mining,Text intelligence, information retrieval and information systems.
Abstract:
In general, there are two types of feedback documents: positive feedback documents and negative feedback documents. Term-Ââ€based approaches can extract many features in text documents, but most include noise. It is clear that all feedback documents contain some noise knowledge that affects the quality of the extracted features. The amount of noise is different from document to another. Therefore, reducing the noise data in the training documents would help to reduce noise in the extracted features. Moreover, we believe that removing some training documents (documents that contain more noise data than useful data) can help to improve the effectiveness of a classifier. Based on that observation, we found that short documents are more important than long documents. Testing that idea, we found that using the advantages of short training documents to improve the quality of extracted features can give a promising result. Moreover, we found that not all training documents are useful for training the classifier.
Azamat Kibekbaev
Ozyegin University, Turkey
Title: BENCHMARKING REGRESSION ALGORITHMS FOR INCOME PREDICTION MODELING
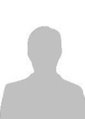
Biography:
Azamat Kibekbaev received his B.S. (2011) from Fatih University and M.S. (2013) from same University both in Industrial Engineering, and doing his Ph.D. (2013) in Industrial Engineering at Özyeğin University. He is particularly interested in data mining applications in banking and healthcare analytics.
Abstract:
This paper aims to predict incomes of customers for banks. In this large-scale income prediction benchmarking paper, we study the performance of various state-of-the-art regression algorithms (e.g. ordinary least squares regression, beta regression, robust regression, ridge regression, MARS, ANN, LS-SVM and CART, as well as two-stage models which combine multiple techniques) applied to five real-life datasets. A total of 16 techniques are compared using 10 different performance measures such as R2, hit rate and preciseness etc. It is found that the traditional linear regression results perform comparable to more sophisticated non-linear and two-stage models. The experiments also indicated that many regression techniques yield performances which are quite competitive with each other (as MARS, M5P, ANN and LSSVM).
Wan Sik Nam
Korea University, South Korea
Title: Wafer Yield Prediction based on Virtual Metrology-Generated Variables
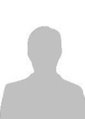
Biography:
Wan Sik Nam was born in Seoul, Republic of Korea, He is currently pursuing the B.S. degree from the School of Industrial Management Engineering, Korea University and working for the Samsung Electronics company as a semiconductor manufacturing engineer. His research interests include the yield prediction model of a semiconductor product that is capable of predicting the wafer yield using the variables generated from the virtual metrology in the semiconductor manufacturing.
Abstract:
Wan Sik Nam was born in Seoul, Republic of Korea, He is currently pursuing the B.S. degree from the School of Industrial Management Engineering, Korea University and working for the Samsung Electronics company as a semiconductor manufacturing engineer. His research interests include the yield prediction model of a semiconductor product that is capable of predicting the wafer yield using the variables generated from the virtual metrology in the semiconductor manufacturing.
Arvind Pandiyan
University of Texas, USA
Title: Improving Similarity Search In Irregular Time-Series Using Dynamic Time Warping
Biography:
Arvind pandiyan is currently pursuing his MS in Computer Science in UT, Dallas and has graduated from PES institute of Technology in the year 2014. He has research interests in Data Mining, Machine learning and Big Data Analysis.
Abstract:
Abstract: Dynamic Time Warping (DTW) is one of the prevailing distance measures used in time-series, though it is computationally costly. DTW is providing optimal alignment between two time-series. The time-series show similarity and DTW exploits the existence of similarity. In this paper, we present techniques that can be employed to improve similarity search in irregular time series data. The drawbacks in the classical approach of converting the irregular time series to a regular one before the similarity search techniques are identified and appropriate solutions for overcoming them are implemented. Simulations with real and synthetic data sets reveal that the proposed techniques are performing well with irregular time series data sets.
Bhabani Shankar Prasad Mishra
KIIT University, India.
Title: An Experimental Study of Parallel Multi-objective Genetic Algorithms
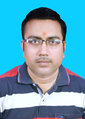
Biography:
Dr. Bhabani Shankar Prasad Mishra is working as an Associate Professor in School of Computer Engineering at KIIT University, Bhubaneswar, Odisha since 2006. He has received the B.Tech in Computer Science in 2003 with Honour’s and distinction, and completed M.Tech in 2005. In M.Tech he has received Gold and Silver Medal from the University. He has received his PhD degree in Computer Science from F.M.University, Balasore, Odisha in 2011. He completed his Post Doctoral Research in Soft Computing Laboratory, Yonsei University, Seoul, South Korea under the Technology Research Program for Brain Science through the National Research Foundation, Ministry of Education, Science and Technology, South Korea. His research interest includes Evolutionary Computation, Neural Networks, Pattern Recognition, Data warehousing and Data Mining, and Big Data. He has already published about 30 research papers in referred journals and conferences, has published one book and edited two books in his credit. He is also acting as an editorial member of various journals.
Abstract:
Many of the optimization problems in the real world are multi-objective in nature, and non-dominated Sorting Genetic Algorithm (NSGA II)is commonly used as a problem solving tool.However, multi-objective problems with non-convex and discrete Pareto front can take enormous computation time to converge to the true Pareto front. Hence, the classical NSGA II (i.e., non- Parallel NSGA II) may fail to solve in ï¥-tolerable amount of time.In this context, we can argue that parallel processing techniquescan be a suitable tool of choice to overcome this difficulty. In this paper we study three different modelsi.e., trigger, island,andcone separation to parallelize NSGA-IIto solve multi-objective 0/1 knapsack problem. Further,we emphasize on two factors that can scale the parallelism i.e., convergence and time. The experimental results confirm that cone separation model is showing a clear edge over trigger and island models in terms of processing time and approximation to the true Pareto front.
- Data Mining Tasks and Processes
Location: USA
Session Introduction
Podili V S Srinivas
Professor
Title: Mining Big Data: Current Status, and Dynamic Energy Management using Big Data Analytics.
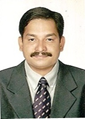
Biography:
Dr. Podili.V.S.Srinivas is working as a Professor in the Department of Computer Science & Engineering, at Gokaraju Rangaraju Institute of Engineering & Technology, Hyderabad, Telangana. He has obtained his Ph.D in Computer Science and Engineering in the area of Computer Networks from JNTUH, Hyderabad in the year 2009. He obtained his M.Tech from JNTUH, Hyderabad in 2003 and obtained his Graduation from Institution of Engineers (India) in 1990. He has a rich experience of total 23 years, out of which 2 years of Industry and 21 Years of academic. Professor Srinivas has published 72 research papers in different refereed International journals and conferences in India as well as abroad. His areas of interests include Computer Networks, Cloud Computing, Big Data and IoT. He delivers many tutorial talks, invited talks and also presented research papers in many International Conferences and Workshops.
Abstract:
Dr. Podili.V.S.Srinivas is working as a Professor in the Department of Computer Science & Engineering, at Gokaraju Rangaraju Institute of Engineering & Technology, Hyderabad, Telangana. He has obtained his Ph.D in Computer Science and Engineering in the area of Computer Networks from JNTUH, Hyderabad in the year 2009. He obtained his M.Tech from JNTUH, Hyderabad in 2003 and obtained his Graduation from Institution of Engineers (India) in 1990. He has a rich experience of total 23 years, out of which 2 years of Industry and 21 Years of academic. Professor Srinivas has published 72 research papers in different refereed International journals and conferences in India as well as abroad. His areas of interests include Computer Networks, Cloud Computing, Big Data and IoT. He delivers many tutorial talks, invited talks and also presented research papers in many International Conferences and Workshops.
- Data Mining Tools and Software
Location: USA
Session Introduction
Dike O.A.
Akanu Ibiam Federal Polytechnic, Nigeria
Title: The Effects of Square Root Transformation on a Gamma Distributed Error Component of a Multiplicative Error Model(MEM)
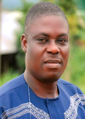
Biography:
Dike O.A. has completed his M.Sc at the age of 39 years in Statistics from Abia State University, Uturu, and a Doctoral student in Statistics at Abia State University, Uturu. He is the Head of Department of Mathematics/Statistics in Akanu Ibiam Federal Polytechnic, Unwana, Nigeria. He has published more than 10 papers in reputed journals and is currently serving as a reviewer in Central Bank of Nigeria (CBN) Journal of Applied Statistics and a member Editorial Board of School of Science Journal.
Abstract:
In this paper, we studied the effect of square root transformation on a Gamma distributed error component of a multiplicative error model with mean 1.0with a view to establishing the condition for the successful transformation. The probability density function (pdf), first and second moments of the square root transformed error component (et*) were established. From the results of the study, it was found that the square root transformed error component was normal with unit mean and variance, approximately1/4times that of the original error (et) before transformation except when the shape parameter is equal to one. However, Anderson Darling’s test for normality on the simulated error terms confirmed normality for et* at (P<0.05). These showed that the square root transformation normalizes a non-normal Gamma distributed error component. Finally, numerical illustrations were used to back up the results established. Thus, a successful square root transformation is achieved when 1/4ï³2< 1.0 which implies that ï³2ï‚£ ¼.
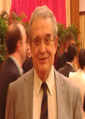
Biography:
Prof. Sousa has more than 40 years of engineering experience. He has an extensive international experience on a large range of projects. He is Full Professor at the University of Porto in Portugal and is multilingual. He has authored or co-authored over 20 books and hundreds of journal articles, presentations and reports. He was President of SKEC Engineering Consulting and is consultant for Laboratory of Deep Underground Engineering, Beijing and consulting engineer in Switzerland, China, Oman, and Portugal. He is now professor at China University of Mining and Technology, Beijing, and Sichuan University, Chengdu, China.
Abstract:
In underground works engineering rockburst is characterized by a violent explosion of a rock block causing a sudden rupture in the rock and is quite common at high depths and every year is responsible for many accidents worldwide. It is critical to understand the phenomenon of rockburst, focusing on the patterns of occurrence so these events can be avoided and/or managed saving costs and possibly lives. The failure mechanism of rockburst needs to be better understood. Laboratory experiments are undergoing at the Laboratory for Geomechanics and Deep Underground Engineering of Beijing. A large number of rockburst tests were performed and their information collected, stored in a database and analyzed. Data Mining (Multiple Regression, Artificial Neural Networks and Support Vector Machines) techniques were applied to the database in order to develop predictive models for the rockburst maximum stress (σRB) and rockburst risk index (IRB). These indexes are very important in rockburst prediction and characterization. The database was composed by 139 laboratory rockburst tests. The results for σRB emphasized the importance of the uniaxial compressive strength of the rock and the horizontal in situ stresses. All the developed models presented excellent results, however the model based on the Support Vector Machines algorithm presents the best performance. The models developed for IRB presented excellent results when the Artificial Neural Network algorithm was used. With the developed models it is possible to predict these parameters with high accuracy levels using data from the rock mass and specific project.
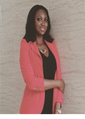
Biography:
Ogunjobi, Olivia Abiola is a Senior Business Analyst with Dangote group. A B.Sc Statistics graduate from University of Ilorin, Kwara State, Nigeria. She is currently working on the business strategy of a new project-Innovation (a new brand). She has been with Dangote Group since 2009. She possess excellent numeric skills, ability to multi task, innovative, target oriented Excellent communication and interpersonal relationship skills, articulate and very effective working with people of different backgrounds and temperament. She is very hard working and delivers on the job within timelines.
Abstract:
Data mining tools are software components and theories that allow users to extract information from data. The tools provide individuals and companies with the ability to gather large amounts of data and use it to make determinations about a particular user or groups of users. Some of the most common uses of data mining tools are in the fields of marketing, Sales, fraud protections and surveillance. The manual extraction of data has existed for hundreds of years. However, the automation of data mining has been most prevalent since the dawn of the computer age. During the 20th century, various computer sciences emerged to help support the concept of developing data mining tools. The overall goal of the utilization of the tools is to uncover hidden patterns. For example, if a marketing company finds that a person takes a monthly trip from New York City to Los Angeles, it becomes beneficial for that company to advertise details of the destination to the individual. Data mining is a process that analysis large amount of data to find new and hidden information that improves business efficiency and it is used to gain competitive advantage and helps the business grow. To predict market trend. Used to analyse shopping pattern within stores based on POS (Point of Sales) information and it is used to answer questions like: how much our customer is likely to spend over a period of time, to know the frequency of customer purchasing behaviour. To know the best type of advert to use to market our product. To know the most effective means of advertisement. And it helps us to improve decision making process which has led to improved efficiency in inventory management and financial forecasting. Data mining tools also helps to determine the business trend of the company, it also helps in Planning: Budgeting &forecasting, on the overall it will enhance business growth and profitability.
Ahmed N. AL-Masri
American University in the Emirates, UAE
Title: Implementing Machine Learning for Big Data Analytics, Challenges and Solutions
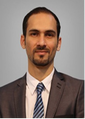
Biography:
Dr. Ahmed AL-Masri received his Ph.D degree in the field of Artificial Intelligence application from University Putra Malaysia. He has more than 6 years’ experience in teaching, programming and research. He involved in many project used artificial neural network system such as forecasting, online monitoring, smart grid, security assessment in electrical power system and dynamic system stability. He is acting as a reviewer for various International and National Journals, and Member of the Institute of Electrical and Electronic Engineers (IEEE). His professional expertise is in the design, analysis of artificial intelligence system, security assessment, parallel processing, virtualization, cloud computing and system automation.
Abstract:
Big Data analytics is one of the great trials in Learning Machine algorithms, as most of real life applications include a massive information or big data knowledge base. On the other hand, the artificial intelligent system with data knowledge base should be able to compute the result in very accurate and fast manner. This paper focused on the challenges and solutions of using Learning Machine with Big Data. Data processing is a mandatory step to transform Big Data which is unstructured into meaningful and optimized data set in any LM module. However, it is necessary to deploy an optimized data set to support the distributed processing and real-time application. This work also reviewed the current used technologies on the Big Data analysis and LM computation. The revision emphasizes on the viability of using different solution for a certain applications could increase the performance of LM. The new development especially in cloud computing and data transaction speed gives more advantages to the practical use of artificial intelligence applications.
Dominik Slezak
University of Warsaw, Poland
Title: Knowledge Pit Platform for Modern Data Mining Competitions
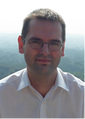
Biography:
Dominik Ślęzak received Ph.D. in 2002 from University of Warsaw and D.Sc. in 2011 from Polish Academy of Sciences. In 2005 he co-founded Infobright Inc., where he holds position of chief scientist. He is also associate professor at Institute of Mathematics, University of Warsaw. He delivered invited talks at over 20 international conferences. He is co-author of over 150 papers and co-inventor in 5 granted US patents. He serves as associate editor for several scientific journals. In 2014 he served as general program chair of IEEE/WIC/ACM Web Intelligence Congress. In 2012-2014 he served as president of International Rough Set Society.
Abstract:
We outline current development of the Knowledge Pit platform (knowledgepit.fedcsis.org) aimed at organization of online data mining competitions. We summarize competitions held so far and planned for the nearest future. We discuss how assumptions about characteristics of complex classification problems and their modern solutions have affected the architecture of our platform, with respect to the size and dimensionality of considered data sets, comparative evaluation of submitted classifiers and their ensembles, as well as final utilization of the best submitted solutions in practice. As case studies, we investigate data-mining-related challenges emerging in our current research projects concerning risk management in coal mines and fire&rescue actions.
- Data Warehousing